Budgeting for AI: How Much Do I Need?

Admin
4th July 2024
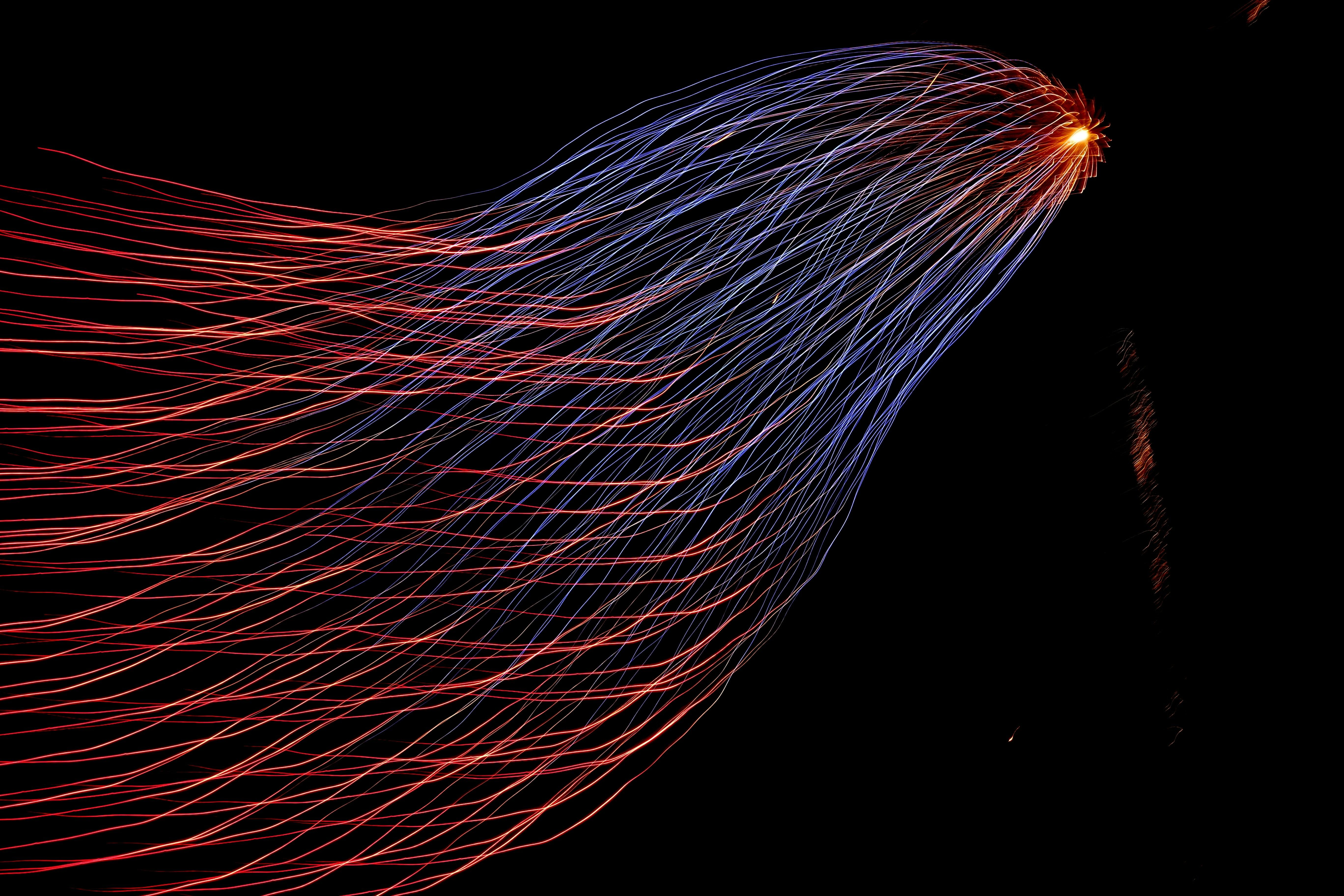
Introduction
Artificial Intelligence is rapidly transforming industries by automating processes, enhancing decision-making, and uncovering new insights. As with any significant technological investment, AI requires careful budgeting to ensure resources are utilized efficiently and the return on investment (ROI) is maximised. This blog post will guide you through the essential aspects of budgeting for AI, from starting small with pilot projects to scaling up while avoiding common financial pitfalls.
Importance of Budgeting for AI
Budgeting for AI is crucial because it helps organisations manage costs, allocate resources effectively, and ensure that AI initiatives align with business goals. Without a clear budget, AI projects can quickly become expensive experiments with unclear outcomes. A well-planned budget ensures that AI investments deliver value and contribute to the organisation's overall strategy.
Development Costs
AI development costs include salaries for data scientists, AI engineers, and developers. These professionals design, build, and fine-tune AI models, which can be a labour-intensive and time-consuming process. Ensuring you have a skilled team is fundamental to the success of your AI initiatives.
• Example: A mid-sized company might budget $150,000 to $200,000 annually for a senior data scientist, $120,000 to $160,000 for an AI engineer, and $90,000 to $120,000 for a machine learning developer. (US salary)
Infrastructure Costs
Infrastructure costs encompass the hardware and software required to support AI development and deployment. This includes servers, GPUs for training deep learning models, cloud services, and data storage solutions. Investing in robust infrastructure is essential to handle the computational demands of AI.
• Example: An organization may invest $100,000 to $500,000 in high-performance computing (HPC) infrastructure or opt for cloud services like AWS, Google Cloud, or Azure, which offer scalable pricing models. For instance, using NVIDIA's A100 GPU on AWS can cost around $40,000 per year, depending on usage.
Software and Tools
AI development requires various software tools, including frameworks, libraries, and platforms. While some tools are open-source and free, others may require licensing fees. Selecting the right combination of tools is critical to building effective AI solutions.
• Example: Tools like TensorFlow and PyTorch are popular open-source options. Commercial platforms like INTELLITHING offer more advanced features but can cost upwards of $50,000 annually.
Training Data
High-quality training data is the cornerstone of effective AI models. Costs associated with acquiring, processing, and labeling data can be significant. Organizations may need to purchase data or invest in data annotation services to ensure their models are trained on accurate and relevant information.
• Example: Acquiring labeled data can cost between $1,000 and $10,000 per 1,000 labels, depending on the complexity. Data annotation services like Amazon Mechanical Turk or Scale AI offer scalable solutions.
Maintenance and Support
AI systems require ongoing maintenance and support to stay relevant and effective. This includes updating models with new data, fixing bugs, and adapting to changing business needs. Maintenance costs should be factored into the long-term budget.
• Example: Annual maintenance costs can range from 15% to 20% of the initial development cost. If an AI project initially costs $500,000, expect to spend $75,000 to $100,000 annually on maintenance.
Consulting and Training
Many organizations seek external expertise to jumpstart their AI initiatives. Consulting services can provide valuable guidance and training to help internal teams build their capabilities. While this can be an additional cost, it often accelerates the development process and ensures best practices are followed.
• Example: AI consulting firms may charge between $200 to $500 per hour. Investing in a week-long workshop could cost $20,000 to $50,000 but significantly enhance the team's capabilities.
Starting Small
Pilot Projects and Proof of Concept
Starting with a pilot project or proof of concept (PoC) is a prudent approach to AI budgeting. These small-scale projects help validate the feasibility and potential of AI solutions before committing significant resources.
• Example: A retailer might develop a PoC to implement an AI-driven inventory management system, costing around $50,000 to $100,000, to reduce stockouts and overstock.
Benefits of Starting with a Small Budget
Starting with a small budget offers several advantages:
• Minimized Risk: Smaller investments reduce financial risk, making it easier to manage setbacks and learn from mistakes without significant financial repercussions.
• Learning Opportunity: Pilot projects provide valuable learning opportunities. Teams can experiment with different AI techniques, tools, and approaches to find what works best for their specific needs.
• Quick Wins: Early successes in pilot projects can build momentum and support within the organization. Demonstrating tangible benefits from AI can help secure additional funding and buy-in from stakeholders.
Examples of Low-Cost AI Projects
• Chatbots: Implementing AI chatbots for customer service can improve response times and customer satisfaction. Chatbots can handle routine inquiries, freeing up human agents for more complex issues. Costs can range from $10,000 to $30,000 for basic chatbot development.
• Predictive Analytics: Using AI for demand forecasting or sales predictions can help organizations optimize inventory levels, reduce waste, and improve overall efficiency. A small project might cost $20,000 to $50,000.
• Image Recognition: Simple image classification tasks, such as identifying defects in manufacturing or managing inventory, can provide quick wins and demonstrate AI’s potential. Such projects can start at $15,000 to $40,000.
Testing the Hypothesis
Methods for Testing AI Solutions
Testing AI solutions involves evaluating their performance against predefined metrics. Several methods can be used to test AI solutions effectively:
• A/B Testing: Comparing AI-driven processes with traditional methods can help measure the effectiveness of AI. For instance, a company might compare sales generated by an AI-powered recommendation engine versus a human-curated one.
• Pilot Programs: Implementing AI in a controlled environment, such as a specific department or process, allows for detailed performance evaluation. This approach helps identify potential issues and areas for improvement.
• Simulation: Using historical data to simulate AI performance can provide insights into how the AI solution might perform in real-world scenarios. This method is particularly useful for predictive analytics and financial modeling.
Measuring Success and ROI
Establishing key performance indicators (KPIs) and ROI metrics is essential for measuring the success of AI projects. Common metrics include:
• Accuracy and Precision: For predictive models, accuracy and precision are critical indicators of how well the AI performs.
• Time Savings: Measuring the reduction in time taken for tasks can demonstrate the efficiency gains from AI implementation.
• Cost Savings: Reductions in operational costs, such as labour or material savings, provide a clear financial benefit.
• Revenue Growth: Increases in sales or profit margins can indicate the direct impact of AI on the organization’s bottom line.
Adjusting the Budget Based on Results
Based on the outcomes of initial tests, adjust the budget to either scale up successful initiatives or reconsider projects that did not meet expectations. Continuous evaluation and adjustment are key to effective AI budgeting. If a pilot project shows promising results, allocate additional resources to expand its scope. Conversely, if the project fails to deliver the expected benefits, reallocate funds to other initiatives or refine the approach before investing further.
Scaling Up
When and How to Increase the Budget
Once pilot projects demonstrate clear value and alignment with business goals, it’s time to consider scaling up. Increase the budget when:
• Successful Proof of Concept: The pilot project achieves its objectives and delivers measurable benefits.
• Alignment with Business Goals: The AI initiative aligns with the organization’s strategic priorities and has the potential to drive significant impact.
• Stakeholder Support: Key stakeholders support the initiative and are willing to invest in its expansion.
To increase the budget effectively, consider:
• Expanding Successful Projects: Invest more in projects with proven ROI. For example, if an AI-powered recommendation engine boosts sales, scale it up across additional product lines or markets.
• Broadening Scope: Apply AI to other areas of the business. If AI has improved customer service, explore its potential in areas like supply chain management or marketing.
• Enhancing Infrastructure: Upgrade hardware and software to support larger-scale AI deployments. This may involve investing in more powerful servers, additional data storage, or advanced AI platforms.
Successful Scaling Strategies
Scaling AI requires a strategic approach to manage complexity and ensure sustainable growth. Consider the following strategies:
• Phased Approach: Gradually increase investment to manage risk. Start with a limited scale-up and expand further based on initial results.
• Cross-Functional Teams: Involve various departments to ensure comprehensive integration. Cross-functional teams can provide diverse perspectives and ensure AI solutions address different business needs.
• Agile Methodology: Use iterative development to refine AI solutions continuously. An agile approach allows for quick adjustments based on feedback and changing requirements.
Long-Term Cost Planning
Plan for long-term costs, including maintenance, upgrades, and potential retraining of AI models. Consider a multi-year budget that accounts for these ongoing expenses. Long-term planning should also include investments in upskilling employees, staying updated with technological advancements, and adapting to evolving business needs.
Avoiding Overspending
Common Pitfalls in AI Budgeting
Avoiding overspending is critical to maximizing the ROI of AI investments. Common pitfalls include:
• Underestimating Complexity: Failing to account for the complexities of AI development and integration can lead to cost overruns. Ensure that your budget includes adequate resources for addressing technical challenges.
• Lack of Clear Goals: Investing in AI without specific objectives can lead to wasted resources. Clearly define the goals and expected outcomes of AI projects to guide budgeting decisions.
• Ignoring Maintenance Costs: Overlooking the need for ongoing support and updates can result in unplanned expenses. Factor in maintenance costs from the outset to avoid budget surprises.
Tips for Cost-Effective AI Implementation
Implementing AI cost-effectively requires careful planning and strategic decision-making. Consider the following tips:
• Leverage Open-Source Tools: Utilize free AI frameworks and libraries to reduce software costs. Open-source tools like TensorFlow and PyTorch provide robust capabilities without licensing fees.
• Cloud Services: Use cloud-based solutions to reduce infrastructure costs. Cloud services offer scalable computing resources, allowing you to pay only for what you use.
• In-House Training: Build internal expertise to reduce reliance on external consultants. Investing in training for your team can lead to long-term cost savings and greater control over AI projects.
• Modular Development: Develop AI solutions in modular components to facilitate easy updates and scalability. A modular approach allows you to enhance or replace individual components without overhauling the entire system.
Conclusion
Budgeting for AI is a dynamic process that requires careful planning, ongoing evaluation, and flexibility. By starting small, testing thoroughly, and scaling responsibly, organizations can harness the power of AI without breaking the bank. Avoiding common pitfalls and implementing cost-effective strategies will ensure that AI investments deliver lasting value and drive business success. As AI continues to evolve, maintaining a proactive and adaptive approach to budgeting will be key to staying ahead of the curve and maximizing the benefits of this transformative technology.
Table of Contents
Subscribe our newsletter

INTELLITHING is the enterprise platform for technical teams to build AI products with no code.

INTELLITHING is the enterprise platform for technical teams to build AI products with no code.
INTELLITHING Offices
Copyright © 2024 INTELLITHING. All Rights reserved.